Seed funding from Elizabeth Blackwell Institute enabled three researchers to develop their projects by including the ideas and experience of patients and the public. Article by Barney Marsh, originally published here. (more…)
How cakes can support charity, community and collaboration
Earlier this year we began to hold monthly bake sales to raise money for charity and to provide a new social space to engage with colleagues. Siân Harris explains.
Involving the public in research: taking the mobile lab on the road
Amy Campbell, Hannah Sallis & Robyn Wootton
How do you feel about researchers tracking your mood using technology? How about tracking your physical activity or your sleep? How much technology will we let into our lives in the name of research?
These were the questions being asked this summer, when a team of researchers (from Bristol, Manchester, London and Oslo) went to speak to members of the public. They took the University of Bristol’s mobile lab around the city of Bristol and surrounding areas, to festivals, community groups and local parks. They wanted to understand public perceptions towards the use of technology to monitor mood and behaviour throughout the day, whether or not this was acceptable, and how to involve a diverse group of people in their research. In this blog post they tell us about what they did and what they found out. (more…)
Why engagement about women’s health needs to go beyond those directly involved
Gemma Sharp and Flo Martin reflect on the importance of engaging with men, as well as women, when it comes to public engagement about menstruation and pregnancy research (more…)
The white tigers of Rewa and Clifton: Mendelism and Bristol Zoo
On 20-21 July we will be welcoming people to Bristol and online around the world to our Mendel at 200 conference. For those who will be joining us face-to-face, there is an opportunity to visit the historic Bristol Zoo Gardens. George Davey Smith shares the story of some of the early zoo residents and how they relate to Mendel’s discoveries. (more…)
From a love of puzzles to studies on BMI – what Mendel’s legacy means to me, and to my cat
In the first of a series of blog posts celebrating 200 years since the birth of Gregor Mendel, Lavinia Paternoster shares how learning about genetics at school shaped her future career – and introduces us to a cat called Mendel (more…)
Why siblings are interesting for genome-wide association studies
Neil Davies discusses a new paper on a genome-wide association study of almost 180,000 siblings and discusses what additional insight siblings bring to such studies. (more…)
Webinar on 19th May honours the career of “Aspirin Man”
In the 1970s two randomised trials of aspirin led by Professor Peter Elwood from the MRC Epidemiology Unit, South Wales made the headlines for finding that a low dose of aspirin had beneficial effects for patients who had had a heart attack.
This was just one of many important discoveries from over 50 years of epidemiological research carried out in South Wales in MRC units, including the Epidemiology Unit initially directed by Archie Cochrane, and then by Peter Elwood. (more…)
Erasing the stain: Challenging the stigma of opioid substitution treatment. Findings from a stakeholder workshop
Author: Vicky Carlisle. Twitter: @Vic_Carlisle, Email: vicky.carlisle@bristol.ac.uk
On Wednesday 7th July 2021, I brought together key stakeholders with an interest in improving opioid substitution treatment (OST) from across the UK. This included people with lived experience, Public Health England staff, local authority public health practitioners, treatment service leads, pharmacists and academics. We discussed the findings of my recently completed PhD, and together we considered the next stages of developing an intervention to improve OST.
A summary of my research
For those not familiar with the topic, OST refers to the treatment of opioid dependency with either methadone or buprenorphine (alongside psychosocial support). Through my research, I wanted to understand what the key facilitators and barriers are to people ‘recovering’ in OST. To do this, I drew on both quantitative and qualitative methodologies. I found that loneliness, isolation and experiences of trauma and stigma were key barriers to recovery; whereas positive social support, discovering a sense of purpose and continuity of care were valuable facilitators.
Importantly, some factors appear to act as both facilitators and barriers to recovery in OST. For instance, I found that some service users used isolation as a form of self-protection (to shield themselves from negative influences), however this often left them feeling lonely and disconnected from the potential benefits offered by developing more positive social support networks.
Undoubtedly, the strongest barrier to recovery was stigma. Service users told me that they experience stigma from a range of sources, including from family and friends, healthcare professionals and members of the wider community. I found similar patterns in the literature review that I carried out (Carlisle et al, 2020). Stigma is like a stain where an individuals’ entire identity is defined by a single, negative attribute. In the case of OST, individuals may possess overlapping stigmatised identities of ‘OST service user’, ‘drug user’ and ‘injecting drug user’. Some will be further stigmatised due to experiencing homelessness, being HIV or Hepatitis C positive or through involvement in sex-work.
“I found that loneliness, isolation and experiences of trauma and stigma were key barriers to recovery”
Community pharmacies are one environment where service users report experiencing a great deal of stigma. Unlike customers collecting other prescriptions, many OST service users receive their medications (methadone/buprenorphine) through an arrangement known as ‘supervised consumption’. This means they must be observed taking their medication by a pharmacist to ensure that it is not diverted to others. This is often conducted in full view of other customers, despite guidelines which recommend that this takes place in a private room or screened area. This leaves OST service users open to the scrutiny of the ‘public gaze’.
My findings have several implications in relation to stigma. Firstly, OST service users receive poorer care than other members of society in healthcare settings, which may result in them avoiding seeking help from drug treatment and for other health conditions. Secondly, stigmatising OST service users makes community re-integration extremely challenging and this has been directly linked to individuals returning to drug using networks as it is somewhere they feel a sense of belonging. The ultimate impact of being repeatedly exposed to stigma is an internalisation of these judgements, resulting in feelings of shame and worthlessness – again impacting on individuals’ ability to seek help and develop supportive new relationships with others.
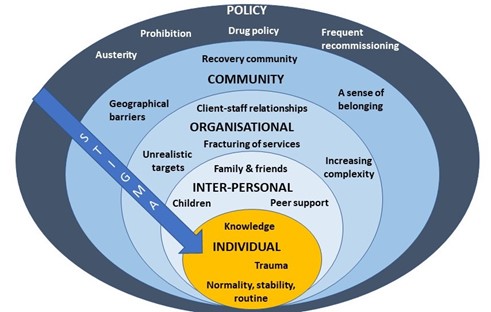
What we discussed during the workshop
Being able to present these findings to key stakeholders was a real highlight of my PhD work; it’s not often that you have the ear of so many invested and engaged individuals in one ‘room’ (albeit a Zoom room!). The findings of my PhD chimed closely with the experiences of those in the room and would be further reflected the next day when Dame Carol Black’s Review of Drugs Part 2 was published, which made specific reference to stigma.
After I presented a short overview of my PhD findings, attendees spent time in small groups discussing how we might address OST stigma at each level of the socioecological system (see figure 1, above). A common thread that ran through each of the groups’ discussions was the importance of embedding interventions within trauma-informed frameworks. Attendees felt that increasing others’ understanding of the impact of trauma and ‘adverse childhood experiences’ (ACEs) may be a key mechanism by which to reduce stigma towards OST service users.
Indeed, a recent study found promising results in relation to this – that increasing the public’s awareness of the role of ACEs in substance use reduced stigmatising attitudes towards people who use drugs (Sumnall et al, 2021). Workshop attendees suggested that this outcome could be achieved through trauma-informed training of all individuals who might work with OST service users, such as pharmacists, the police and medical professionals, as well as those who work in healthcare settings, such as receptionists.
At the individual level there was a discussion about the way that stigma trickles down the socioecological system, resulting in self-stigma or internalised stigma. People felt that the best way to reduce this was to tackle stigma higher upstream first.
When thinking about reducing stigma in everyday inter-personal interactions, people highlighted the importance of using non-stigmatising language. For those who are interested (and I think we all should be!) the Scottish Drug Forum has published an excellent guide here.
Some excellent suggestions were made for reducing stigma that individuals experience in organisations such as pharmacies, hospitals and other settings. This is something that Dr Jenny Scott and I discussed in a recent article for the Pharmaceutical Journal (Scott & Carlisle, 2021). One attendee suggested the introduction of positive role-models within organisations who could be an exemplar of positive behaviour for others (a ‘stigma champion’ perhaps?). Training was identified as a key mechanism through which stigma could be reduced in organisations, including through exposure to people who use drugs (PWUD) and OST service users during training programmes. It was stressed however, that this should be carefully managed to ensure that a range of voices are presented and not just ones supporting dominant discourses around abstinence-based recovery.
Suggestions for improving community integration included increasing access to volunteering opportunities – something that people felt has been impacted by reduced funding to recovery services in recent years. It was also suggested that community and faith leaders could be a potential target for education around reducing stigma and understanding the impact of trauma, as these individuals may be best placed to have conversations about stigma with members of their communities.
Finally, there were some thoughtful discussions around the best way to influence policy to reduce stigma. The importance of showing policymakers the evidence-base from previous successful strategies was highlighted. Something that resulted in a lively debate was the issue of supervised consumption with arguments both for and against (this is also relevant at the organisational level). The group summarised that whilst diversion of medications was a risk for some, a blanket approach to supervised consumption and/or daily collections exposes individuals to stigma in the pharmacy, which leaves individuals vulnerable to dropping out of treatment. It was pointed out that supervised consumption policies were quickly relaxed at the start of Covid-19 restrictions – something that appears to have been done safely and with benefits to service users. It was also highlighted that supervised consumption in OST is inherently stigmatising, as users of other addictive drugs with overdose potential, such as other prescribed opioids and benzodiazepines, are not subjected to the same regulations. This sends a clear message to OST service users that they cannot be trusted. Other key suggestions were:
- Communicating with CQCs and Royal Colleges, who may be particularly interested in understanding how people are treated in their services.
- Drawing on existing stigma policies from other arenas e.g. mental health.
- Highlighting the fiscal benefits of reducing stigma to key decision makers.
- Tapping into plans for the new Police and Crime Commissioners, who have a trauma sub-group.
- Linking into work with ADDER areas, which may provide the evidence for ‘what works’.
What next?
I am now planning to apply for further funding to develop an intervention to reduce organisational stigma towards OST service users. The involvement of service users and other key stakeholders will be crucial in every step of that process, so I will be putting together a steering group as well as seeking out collaborations with academics internationally that have expertise and an interest in this area. I was really pleased to see that Dame Carol Black’s second report makes some concrete recommendations around reducing stigma towards people who use drugs. I hope therefore to be able to work with the current momentum to make OST safer and more attractive to those whose lives depend on it.
I’d like to extend my gratitude to all of the attendees at the workshop and to Bristol’s Drug and Alcohol Health Integration Team (HIT) for supporting this event. If you are an individual with lived experience of OST, an academic, or any other stakeholder working in this area and would like to be involved with future developments, please get in touch with me at vicky.carlisle@bristol.ac.uk or find me on Twitter at @Vic_Carlisle.
References
Carlisle, V., Maynard, O., Padmanathan, P., Hickman, M., Thomas, K. H., & Kesten, J. (2020, September 7). Factors influencing recovery in opioid substitution treatment: a systematic review and thematic synthesis. https://doi.org/10.31234/osf.io/f6c3p
Scott, J & Carlisle, V (2021). A pharmacy resolution for 2021: let’s improve the way patients with addiction are treated. The Pharmaceutical Journal. https://pharmaceutical-journal.com/article/opinion/a-pharmacy-resolution-for-2021-lets-improve-the-way-patients-with-addiction-are-treated
Sumnall, H. R., Hamilton, I., Atkinson, A. M., Montgomery, C., & Gage, S. H. (2021). Representation of adverse childhood experiences is associated with lower public stigma towards people who use drugs: an exploratory experimental study. Drugs: Education, Prevention and Policy, 28(3), 227-239. https://doi.org/10.1080/09687637.2020.1820450
This blog was originally posted on the TARG blog on the 1 October 2021.
COVID-19: Pandemics and ‘Infodemics’
Drs Luisa Zuccolo and Cheryl McQuire, Department of Population Health Sciences, Bristol Medical School, University of Bristol.
The problem
Soon after the World Health Organisation (WHO) declared COVID-19 a pandemic on March 11th 2020, the UN declared the start of an infodemic, highlighting the danger posed by the fast spreading of unchecked misinformation. Defined as an overabundance of information, including deliberate efforts to disseminate incorrect information, the COVID-19 infodemic has exacerbated public mistrust and jeopardised public health.
Social media platforms remain a leading contributor to the rapid spread of COVID-19 misinformation. Despite urgent calls from the WHO to combat this, public health responses have been severely limited. In this project, we took steps to begin to understand and address this problem.
We believe that it is imperative that public health researchers evolve and develop the skills and collaborations necessary to combat misinformation in the social media landscape. For this reason, in Autumn 2020 we extended our interest in public health messaging, usually around promoting healthy behaviours during pregnancy, to study COVID-19 misinformation on social media.
We wanted to know:
What is the nature, extent and reach of misinformation about face masks on Twitter during the COVID-19 pandemic?
To answer this question we aimed to:
- Upskill public health researchers in the data capture and analysis methods required for social media data research;
- Work collaboratively with Research IT and Research Software Engineer colleagues to conduct a pilot study harnessing social media data to explore misinformation.
The team
Dr Cheryl McQuire got the project funded and off the ground. Dr Luisa Zuccolo led it through to completion. Dr Maria Sobczyk checked the data and analysed our preliminary data. Research IT colleagues, led by Mr Mike Jones, helped to develop the search strategy and built a data pipeline to retrieve and store Twitter data using customised application programming interfaces (APIs) accessed through an academic Twitter account. Research Software Engineering colleagues, led by Dr Christopher Woods, provided consultancy services and advised on the analysis plan and technical execution of the project.
Cheryl McQuire, Luisa Zuccolo, Maria Sobcyzk, Mike Jones, Christopher Woods. (Left to Right)
Too much information?!
Initial testing of the Twitter API showed that keywords, such as ‘mask’ and ‘masks’, returned an unmanageable amount of data, and our queries would often crash due to an overload of Twitter servers (503-type errors). To address this, we sought to reduce the number of results, while maintaining a broad coverage of the first year of the pandemic (March 2020-April 2021).
Specifically, we:
I) Searched for hashtags rather than keywords, restricting to English language.
II) Requested original tweets only, omitting replies and retweets.
III) Broke each month down into its individual days in our search queries to minimise the risk of overload.
IV) Developed Python scripts to query the Twitter API and process the results into a series of CSV files containing anonymised tweets, metadata and metrics about the tweets (no. of likes, retweets etc.), and details and metrics about the author (no. of followers etc.).
V) Merged data into a single CSV file with all the tweets for each calendar month after removing duplicates.
What did we find?
Our search strategy delivered over three million tweets. Just under half of these were filtered out by removing commercial URLs and undesired keywords, the remaining 1.7m tweets by ~700k users were analysed using standard and customized R scripts.
First, we used unsupervised methods to describe any and all Twitter activity picked up by our broad searches (whether classified as misinformation or not). The timeline of this activity revealed clear peaks around the UK-enforced mask mandates in June and September 2020.
We further described the entire corpus of tweets on face masks by mapping the network of its most common bigrams and performing sentiment analysis.
We then quantified the nature and extent of misinformation through topic modelling, and used simple counts of likes to estimate the reach of misinformation. We used semi-supervised methods including manual keyword searches to look for established types of misinformation such as face masks restricting oxygen supply. These revealed that the risk of bacterial/fungal infection was the most common type of misinformation, followed by restriction of oxygen supply, although the extent of misinformation on the risks of infection decreased as the pandemic unfolded.
Extent of misinformation (no tweets), according to its nature: 1- gas exchange/oxygen deprivation, 2- risk of bacterial/fungal infection, 3- ineffectiveness in reducing transmission, 4- poor learning outcomes in schools.
Relative to the volume of tweets including the hashtags relevant to face masks (~1.7m), our searches uncovered less than 3.5% unique tweets containing one of the four types of misinformation against mask usage.
A summary of the nature, extent and reach of misinformation on face masks on Twitter – results from manual keywords search (semi-supervised topic modelling).
A more in-depth analysis of the results attributed to the 4 main misinformation topics by the semi-supervised method revealed a number of potentially spurious topics. Refinements of these methods including iterative fine-tuning were beyond the scope of this pilot analysis.
Our initial exploration of Twitter data for public health messaging also revealed common pitfalls of mining Twitter data, including the need for a selective search strategy when using academic Twitter accounts, hashtag ‘hijacking’ meaning most tweets were irrelevant, imperfect Twitter language filters and ads often exploiting user mentions.
Next steps
We hope to secure further funding to follow-up this pilot project. By expanding our collaboration network, we aim to improve the way we tackle misinformation in the public health domain, ultimately increasing the impact of this work. If you’re interested in health messaging, misinformation and social media, we would love to hear from you – @Luisa_Zu and @cheryl_mcquire.
Note:
This blog post was original written for the Jean Golding Institute blog