Sarah Lewis, George Davey Smith and Marcus Munafo
Follow Sarah, George and Marcus on Twitter
Due to the SARS-CoV-2 pandemic schools across the United Kingdom were closed to all but a small minority of pupils (children of keyworkers and vulnerable children) on the 20th March 2020, with some schools reporting as few as 5 pupils currently attending. The UK government have now issued guidance that primary schools in England should start to accept pupils back from the 1st June 2020 with a staggered return, starting with reception, year 1 and year 6.
Concern from teachers’ unions
This has prompted understandable concern from the teachers’ unions, and on the 13th May, nine unions which represent teachers and education professionals signed a joint statement calling on the government to postpone reopening school on the 1st June, “We all want schools to re-open, but that should only happen when it is safe to do so. The government is showing a lack of understanding about the dangers of the spread of coronavirus within schools, and outwards from schools to parents, sibling and relatives, and to the wider community.” At the same time, others have suggested that the harms to many children due to neglect, abuse and missed educational opportunity arising from school closures outweigh the small increased risk to children, teachers and other adults of catching the virus.
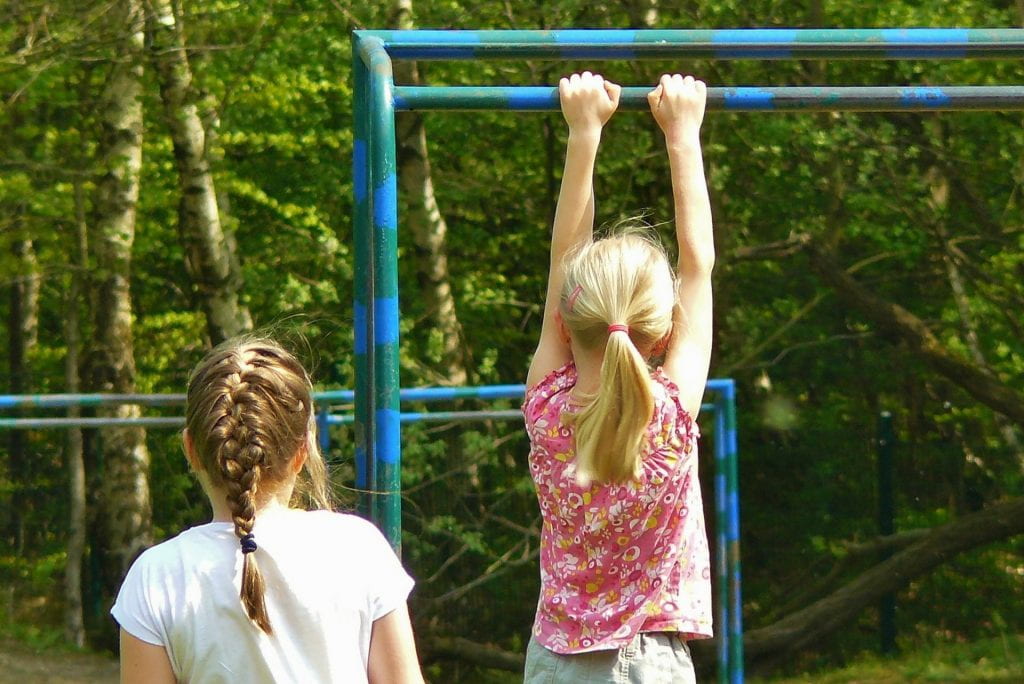
Weighing up the risks to children and teachers
So what do we know about the risk to children and to teachers? We know that children are about half as likely to catch the virus from an infected person as adults, and if they do catch the virus they are likely to have only mild symptoms. The current evidence, although inconclusive, also suggests that they may be less likely to transmit the virus than adults. However, teachers have rightly pointed out that there is a risk of transmission between the teachers themselves and between parents and teachers.
The first death from COVID-19 in England was recorded at the beginning of March 2020 and by the 8th May 2020 39,071 deaths involving COVID-19 had been reported in England and Wales. Just three of these deaths were among children aged under 15 years and only a small proportion of the deaths (4416 individuals, 11.3%) were among working aged people. Even among this age group risk is not uniform; it increases sharply with age from 2.6 in 100,000 for 25-44 years olds with a ten fold increase to 26 in 100,000 individuals for those aged 45-64.
Risks to teachers compared to other occupations
In addition, each underlying health condition increases the risk of dying from COVID-19, with those having at least 1 underlying health problem making up most cases. The Office for National Statistics in the UK have published age standardised deaths by occupation for all deaths involving COVID-19 up to the 20th April 2020. Most of the people dying by this date would have been infected at the peak of the pandemic in the UK prior to the lockdown period. They found that during this period there were 2494 deaths involving Covid-19 in the working age population. The mortality rate for Covid-19 during this period was 9.9 (95% confidence intervals 9.4-10.4) per 100,000 males and 5.2 (95%CI 4.9-5.6) per 100,000 females, with Covid-19 involved in around 1 in 4 and 1 in 5 of all deaths among males and females respectively.
Amongst teaching and education professionals (which includes school teachers, university lecturers and other education professionals) a total of 47 deaths (involving Covid-19) were recorded, equating to mortality rates of 6.7 (95%CI 4.1-10.3) per 100,000 among males and 3.3 (95%CI 2.0-4.9) per 100,000 among females, which was very similar to the rates of 5.6 (95%CI 4.6-6.6) per 100,000 among males and 4.2(95%CI 3.3-5.2) per 100,000 females for all professionals. The mortality figures for all education professionals includes 7 out of 437000 (or 1.6 per 100,000 teachers) primary and nursery school teachers and 17 out of 395000 (or 4.3 per 100,000 teachers) secondary school teachers. A further 20 deaths occurred amongst childcare workers giving a mortality rate amongst this group of 3.4 (95%CI=2.0-5.5) per 100,000 females (males were highly underrepresented in this group), this is in contrast to rates of 6.5 (95%CI=4.9-9.1) for female sales assistants and 12.7(95%CI= 9.8-16.2) for female care home workers.
Covid-19 risk does not appear greater for teachers than other working age individuals
In summary, based on current evidence the risk to teachers and childcare workers within the UK from Covid-19 does not appear to be any greater than for any other group of working age individuals. However, perceptions of elevated risk may have occurred, prompting some to ask “Why are so many teachers dying?” due to the way this issue is portrayed in the media with headlines such as “Revealed: At least 26 teachers have died from Covid-19” currently on the https://www.tes.com website. This kind of reporting, along with the inability of the government to communicate the substantial differences in risk between different population groups – in particular according to age – has caused understandable anxiety among teachers. Whilst, some teachers may not be prepared to accept any level of risk of becoming infected with the virus whilst at work, others may be reassured that the risk to them is small, particularly given that we all accept some level of risk in our lives, a value that can never be zero.
Likely impact on transmission in the community is unclear
As the majority of parents or guardians of school aged children will be in the 25-45 age range, the risk to them is also likely to be small. Questions remain however around the effect of school openings on transmission in the community and the associated risk. This will be affected by many factors including the existing infection levels in the community, the extent to which pupils, parents and teachers are mixing outside of school (and at the school gate) and mixing between individuals of different age groups. This is the primary consideration of the government Scientific Advisory Group for Emergencies (SAGE) who are using modelling based on a series of assumptions to determine the effect of school openings on R0.
Sarah Lewis is a Senior Lecturer in Genetic Epidemiology in the department of Population Health Sciences, and is an affiliated member of the MRC Integrative Epidemiology Unit (IEU), University of Bristol
George Davey Smith is a Professor of Clinical Epidemiology, and director of the MRC IEU, University of Bristol
Marcus Munafo is a Professor of Biological Psychology, in the School of Psychology Science and leads the Causes, Consequences and Modification of Health Behaviours programme of research in the IEU, University of Bristol.