Dr Gemma Sharp and Dr Tim Morris
Follow Gemma and Tim on twitter
The COVID-19 pandemic is proving to be a period of great uncertainty. Will we get it? If we get it, will we show symptoms? Will we have to go to hospital? Will we be ok? Have we already had it?
These questions are difficult to answer because, currently, not much is known about who is more at risk of being infected by coronavirus, and who is more at risk of being seriously ill once infected.
Researchers, private companies and government health organisations are all generating data to help shed light on the factors linked to COVID-19 infection and severity. You might have seen or heard about some of these attempts, like the COVID-19 Symptom Tracker app developed by scientists at King’s College London, and the additional questions being sent to people participating in some of the UK’s biggest and most famous health studies, like UK Biobank and the Avon Longitudinal Study of Parents and Children (ALSPAC).
These valuable efforts to gather more data will be vital in providing scientific evidence to support new public health policies, including changes to the lockdown strategy. However, it’s important to realise that data gathered in this way is ‘observational’, meaning that study participants provide their data through medical records or questionnaires but no experiment (such as comparing different treatments) is performed on them. The huge potential impact of COVID-19 data collection efforts makes it even more important to be aware of the difficulties of using observational data.
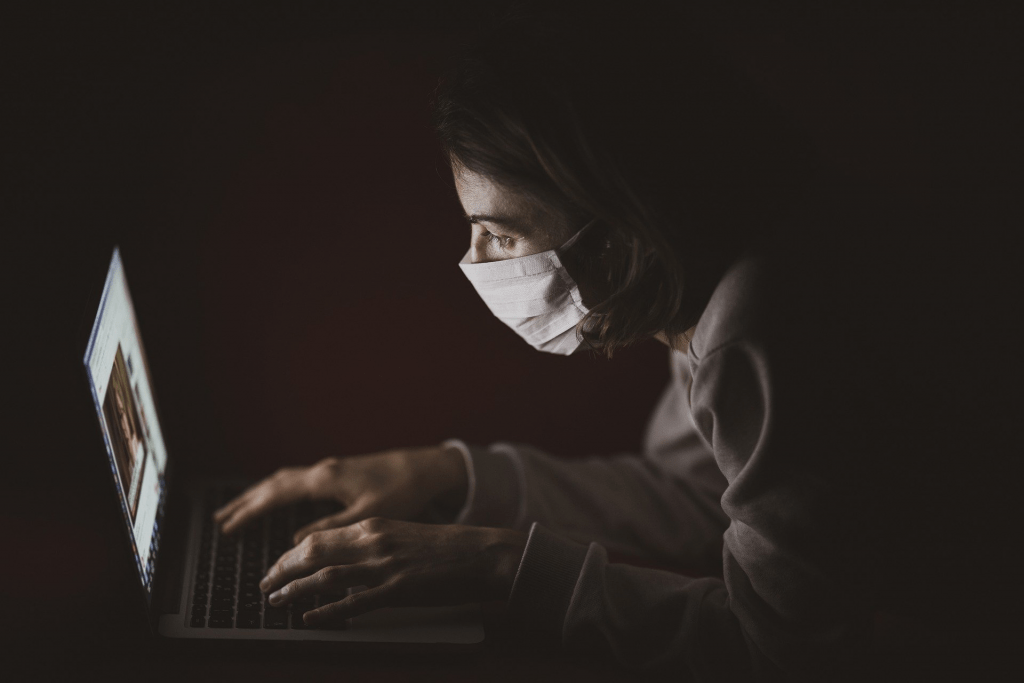
Correlation does not equal causation (the reason observational epidemiology is hard)
These issues boil down to one main problem with observational data: that it is difficult to tease apart correlation from causation.
There are lots of factors that correlate but clearly do not actually have any causal effect on each other. Just because, on average, people who engage in a particular behaviour (like taking certain medications) might have a higher rate of infection or severe COVID-19 illness, it doesn’t necessarily mean that this behaviour causes the disease. If the link is not causal, then changing the behaviour (for example, changing medications) would not change a person’s risk of disease. This means that a change in behaviour would provide no benefit, and possibly even harm, to their health.
This illustrates why it’s so important to be sure that we’re drawing the right conclusions from observational data on COVID-19; because if we don’t, public health policy decisions made with the best intentions could negatively impact population health.
Why COVID-19 research participants are not like everyone else
One particular issue with most of the COVID-19 data collected so far is that the people who have contributed data are not a randomly drawn or representative sample of the broader general population.
Only a small percentage of the population are being tested for COVID-19, so if research aims to find factors associated with having a positive or negative test, the sample is very small and not likely to be representative of everyone else. In the UK, people getting the test are likely to be hospital patients who are showing severe symptoms, or healthcare or other key workers who are at high risk of infection and severe illness due to being exposed to large amounts of the virus. These groups will be heavily over-represented in COVID-19 research, and many infected people with no or mild symptoms (who aren’t being tested) will be missed.
Aside from using swab tests, researchers can also identify people who are very likely to have been infected by asking about classic symptoms like a persistent dry cough and a fever. However, we have to consider that people who take part in these sorts of studies are also not necessarily representative of everyone else. For example, they are well enough to fill in a symptom questionnaire. They also probably use social media, where they likely found out about the study. They almost certainly own a smartphone as they were able to download the COVID-19 Symptom Tracker app, and they are probably at least somewhat interested in their health and/or in scientific research.
Why should we care about representativeness?
The fact that people participating in COVID-19 research are not representative of the whole population leads to two problems, one well-known and one less well-known.
Firstly, as often acknowledged by researchers, research findings might not be generalisable to everyone in the population. Correlations or causal associations between COVID-19 and the characteristics or behaviours of research participants might not exist amongst the (many more) people who didn’t take part in the research, but only in the sub-group who participated. So the findings might not translate to the general population: telling everyone to switch up their medications to avoid infection may only work for some people who are like those studied.
But there is a second problem, called ‘collider bias’ (sometimes also referred to using other names such as selection bias or sampling bias), that is less well understood and more difficult to grasp. Collider bias can distort findings so that certain factors appear related even when there is no relationship in the wider population. In the case of COVID-19 research, relationships between risk factors and infection (or severity of infection) can appear related when no causal effect exists, even within the sample of research participants.
As an abstract example, consider a private school where pupils are admitted only if they have either a sports scholarship or an academic scholarship. If a pupil at this school is not good at sports, we can deduce that they must be good at academic work. This correlation between being poor at sports but being good academically doesn’t exist in the real world outside of this school, but in the sample of school pupils, it appears. And so, with COVID-19 research, in the sample of people included in a COVID-19 dataset (e.g. people who have had a COVID-19 test), two factors that influence inclusion (e.g. having COVID-19 symptoms that were severe enough to warrant hospitalisation, and taking medications for a health condition that puts you at high risk of dying from COVID-19) would appear to be associated, even when they are not. That is, to be in the COVID-19 dataset (to be tested), people are likely to have had either more severe symptoms or to be on medication. The erroneous conclusion would follow that changing one factor (e.g. changing or stopping medications) would affect the other (i.e. lower the severity of COVID-19). Because symptom severity is related to risk of death, stopping medication would appear to reduce the chance of death. As such, any resulting changes to clinical practice would be ineffective or even harmful.
Policymaking is a complex process at the best of times, involving balancing evidence from research, practice, and personal experience with other constraints and drivers, such as resource pressures, politics, and values. Add into that the challenge of making critical decisions with incomplete information under intense time pressure, and the need for good quality evidence becomes even more acute. The expertise of statisticians, who can double check analyses and ensure that conclusions are as robust as possible, should be a central part of the decision making process at this time – and especially to make sure that erroneous conclusions arrived at as a result of collider bias do not translate into harmful practice for people with COVID-19.
*****************************************************************************************************
The main aim of this blog post was to highlight the issue of collider bias, which is notoriously tricky to grasp. We hope we’ve done this but would be interested in your comments.
For those looking for more information, read on to discover some of the statistical methods that can be used to address collider bias….
Now we know collider bias is a problem: how do we fix it?
It is important to consider the intricacies of observational data and highlight the very real problems that can arise from opportunistically collected data. However, this needs to be balanced against the fact that we are in the middle of a pandemic, that important decisions need to be made quickly, and this data is all we have to guide decisions. So what can we do?
There are a few strategies, developed by statisticians and other researchers in multiple fields, that should be considered when conducting COVID-19 research:
- Estimate the extent of the collider bias:
o Think about the profile of people in COVID-19 samples – are they older/younger or more/less healthy than individuals in the general population?
o Are there any unexpected correlations in the sample that ring alarm bells?
- Try to balance out the analysis by ‘weighting’ individuals, so that people from under-represented groups count more than people from over-represented groups.
- Carry out additional analysis, known as ‘sensitivity analysis’, to assess the extent to which plausible patterns of sample selection could alter measured associations.
For those who would like to read even more, here’s a pre print on collider bias published by our team:
Drs. Sharp and Morris,
I’m a bit confused by your discussion of collider bias. My understanding is that this sort of bias occurs when 1) two variables which are unrelated in the general population both cause a third variable and 2) conditioning on this third variable results in a correlation between the first two variables, rendering a biased estimate of some causal effect of interest. I’m clear on what you see as the two variables which may unrelated in the population: having severe symptoms and taking certain medications. What I’m unclear about is what you take the collider variable to be.
You mention how severity of symptoms as well as taking certain medications affects one’s chance of ending up in the sample. Later, you also say that because symptom severity is related to risk of death, collider bias may make it appear that stopping the use of certain medications decreases risk if death. So, it sounds like you’re saying that chance of ending up in the sample and risk of death are both the collider. But that would only be true if they are both the same thing. Are you saying they’re the same thing?
The chance of ending up in the sample and the risk of death are not the same thing. Based on your interpretation, Drs. Sharp and Morris have implied that both are the same thing because:
1. The severity of symptoms affects the chance of ending up in the sample;
2. The taking of certain medications affects the chance of ending up in the sample;
3. The severity of symptoms affects the risk of death;
4. The taking of certain medications affects the risk of death; and
5. The severity of symptoms and the taking of certain medications affect one and only one thing in common.
However, there is no evidence that Drs. Sharp and Morris intended either 4 or 5 to be true. First, the statement that “stopping medication would appear to reduce the chance of death” is not the same as the statement that “stopping medication would reduce the chance of death”. Second, I am not aware of any statement in the post which can be interpreted to the effect of 5.
Instead, Drs. Sharp and Morris provided an example of how one might reach the erroneous conclusion that stopping medication reduces the chance of death from the following [erroneous] premises:
1. The severity of symptoms affects the chance of ending up in the sample;
2. The taking of certain medications affects the chance of ending up in the sample;
3. The severity of symptoms is associated with the taking of certain medications (i.e., collider bias);
4. Changing the taking of certain medications would affect the severity of symptoms (i.e., correlation equals causation);
5. The severity of symptoms is related to the risk of death; and
6. If the taking of certain medications affects the severity of symptoms, and the severity of symptoms is related to the risk of death, then the taking of certain medications reduces the chance of death.
In short, Drs. Sharp and Morris do not appear to have intended for the chance of ending up in the sample and the risk of death are not the same thing. Instead, the paragraph in question functions as an example of how one might reach the erroneous conclusion that stopping medication reduces the chance of death.
Hi Michael, in the symptom & medication example the collider would be participation in the study (whether an app, questionnaire or testing sample). Because we can only analyse the data points that are observed, we automatically condition on study participation, which becomes a collider. A collider is a variable that is influenced by two other variables and it can be helpful to think of them graphically – they ‘collide’ on a Direct Acylcic Graph – see the right hand panel of first figure here: https://catalogofbias.org/biases/collider-bias/.
Symptom severity, use of medications and risk of death all influence participation, so are not the colliders themselves. However, by conditioning on the collider (participation) ,associations between them can become distorted in the sample.
Is that Michael levitt?
Well, People with severe covid symptoms are also quite likely to have an underlying health condition for which they are often likely to take medication. A correlation may then be assumed between taking medication and having severe symptoms of covid, which is most likely incorrect. (Though theoretically possible)
Or, everybody admitted to hospital was wearing clothes, so nudists are most likely covid free, or asymptomatic.
Also, why didn’t you just say 20% of those Parisians obviously pretended they didn’t smoke. That would have saved me a lot of reading.
In the sample analyzed, wouldn’t people on medication be expected to have lower covid symptoms than those not on medications? (For the same reason why healthcare workers would have lower covid symptoms than the general public, as per https://www.medrxiv.org/content/10.1101/2020.05.04.20090506v2)
If so, wouldn’t one conclude that stopping medication would increase (not decrease) symptoms (and risk of death?)
Apologies – I must be missing something pretty basic here