Amanda Hughes, Kaitlin H. Wade, Matt Dickson, Frances Rice, Alisha Davies, Neil M. Davies & Laura D. Howe
Follow Amanda, Kaitlin, Matt, Alisha, Neil and Laura on twitter
Young people with health problems tend to do less well in school than other students, but it has never been clear why. One explanation is that health problems directly damage educational outcomes. In that case, policymakers aiming to raise educational standards might want to focus first on health as a means of improving attainment.
But are there other explanations? What if falling behind in school can affect health, for instance causing depression? Also, many health problems are more common among children from less advantaged backgrounds – for example, from families with fewer financial resources, or whose parents are themselves unwell. These children also tend to do less well in school, for reasons that may have nothing to do with their own health. How do we know if their health, or their circumstances, are affecting attainment?
It is also unclear if health matters equally for education at all points in development, or particularly in certain school years. Establishing how much health does impact learning, when, and through which mechanisms, would better equip policymakers to improve educational outcomes.

Using genetic data helps us understand causality
Genetic data can help us answer these questions. Crucially, experiences like family financial difficulties, which might influence both a young person’s health and their learning, cannot change their genes. So, if young people genetically inclined to have asthma are more absent from school, or do less well in their GCSEs, that would strongly suggest an impact of asthma itself. Similarly, while falling behind in school might well trigger depression, it cannot change a person’s genetic propensity for depression. So, a connection between genetic propensity for depression and worse educational outcomes supports an impact of depression itself. This approach, of harnessing genetic information to better understand causal processes, is known as Mendelian randomization.
To find out more, we investigated links between
- health conditions in childhood and adolescence
- school absence in years 10 & 11
- and GCSE results.
We used data from 6113 children born in the Bristol area in 1991-1992. All were participants of the Avon Longitudinal Study of Parents and Children (ALSPAC), also known as Children of the 90s. We focused on six different aspects of health: asthma, migraines, body mass index (BMI), and symptoms of depression, of attention-deficit hyperactivity disorder (ADHD), and of autism spectrum disorder (ASD). These conditions, though diverse, have two important things in common: they affect substantial numbers of young people, and they are at least in part influenced by genetics.
Alongside questionnaire data and education records, we also analysed genetic information from participants’ blood samples. From this information, we were able to calculate for each young person a summary score of genetic propensity for experiencing migraines, ADHD, depression, ASD, and for having a higher BMI.
We used these scores to predict the health conditions, rather than relying just on reports from questionnaire data. In this way, we avoided bias due to the impact of the young people’s circumstances, or of their education on their health rather than vice versa.
Even a small increase in school absence predicted worse GCSEs.
We found that, for each extra day per year of school missed in year 10 or 11, a child’s total GCSE points from their best 8 subjects was a bit less than half (0.43) of a grade lower. Higher BMI was related to increased school absence & lower GCSE grades.
Using the genetic approach, we found that young people genetically predisposed towards a higher BMI were more often absent from school, and they did less well in their GCSEs. A standard-deviation increase* in BMI corresponded to 9% more school absence, and GCSEs around 1/3 grade lower in every subject. Together, these results indicate that increased school absence may be one mechanism by which being heavier could negatively impact learning. However, in other analyses, we found a substantial part of the BMI-GCSEs link was not explained by school absence. It’s unclear which other mechanisms are at play here, but work by other researchers has suggested that weight-related bullying, and negative effects of being heavier on young people’s self-esteem, could interfere with learning.
*equivalent to the difference between the median (50th percentile) in population and the 84th percentile of the population
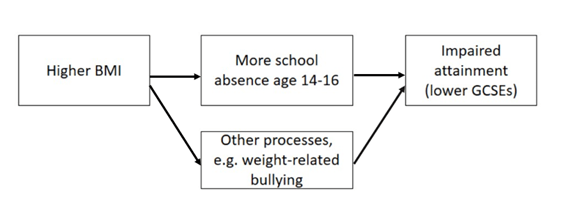
ADHD was related to lower GCSE grades, but not increased school absence.
In line with previous research, young people genetically predisposed to ADHD did less well in their GCSEs. Interestingly, they did not have increased school absence, suggesting that ADHD’s impact on learning works mostly through other pathways. This is consistent with previous research highlighting the importance of other factors on the academic attainment of children with ADHD, including expectations of the school environment, teacher views and attitudes, and bullying by peers.
We found little evidence for an impact of asthma, migraines, depression or ASD on school absence or GCSE results
Our genetic analyses found little support for a negative impact of asthma, migraines, depression or ASD on educational attainment. However, we know relatively little about the genetic influences on depression and ASD, especially compared to the genetics of BMI, which we understand much better. This makes genetic associations with depression or ASD difficult to detect. So, our results should not be taken as conclusive evidence that these conditions do not affect learning.
What does this mean for students and teachers?
Our findings provide evidence of a detrimental impact of high BMI and of ADHD symptoms on GCSE attainment, which for BMI was partially mediated by school absence. When students sent home during the pandemic eventually return to school, the impact on their learning will have been enormous. And while all students will have been affected, our results highlight that young people who are heavier, who have ADHD, or are experiencing other health problems, will likely need extra support.
Further reading
Hughes, A., Wade, K.H., Dickson, M. et al. Common health conditions in childhood and adolescence, school absence, and educational attainment: Mendelian randomization study. npj Sci. Learn. 6, 1 (2021). https://doi.org/10.1038/s41539-020-00080-6
A version of this blog was posted on the journal’s blog site on 21 Jan 2021.
Contact the researchers
Amanda Hughes, Senior Research Associate in Epidemiology: amanda.hughes@bristol.ac.uk